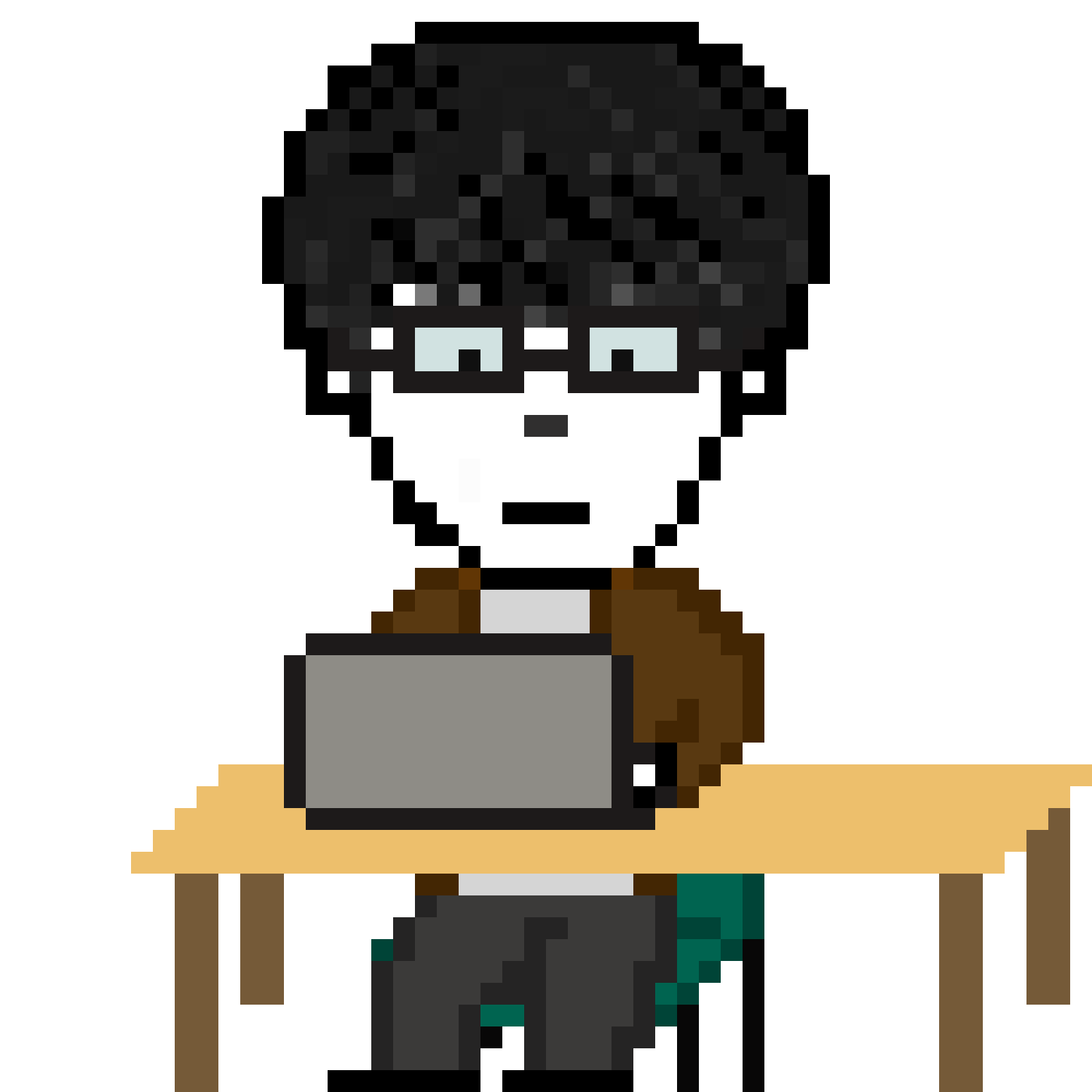
内野 佑基
UCHINO Yuki
連絡先:
yuki.uchino.fe (at) riken.jp
UCHINO, Yuki
CONTACT:
yuki.uchino.fe (at) riken.jp
Profile
名前: | 内野 佑基 | |
メール: | yuki.uchino.fe (at) riken.jp | |
所属: | 理化学研究所 計算科学研究センター | |
住所: | 〒650-0047 兵庫県神戸市中央区港島南町 7-1-26 | |
身長: | りんご14個 | |
体重: | りんご230個 | |
所属学会: |
日本応用数理学会 (2022.07-), 情報処理学会 (2025.04-) |
|
トピック: |
混合精度数値計算, 数値線形代数, 高性能計算, 精度保証付き数値計算 |
Name: | Yuki UCHINO | |
Email: | yuki.uchino.fe (at) riken.jp | |
Affiliation: | RIKEN Center for Computational Science | |
Address: | 7-1-26 Minatojima-minami-machi, Chuo-ku, Kobe, Hyogo 650-0047, Japan | |
Pronoun: | he / him / his | |
Memberships: |
The Japan Society for Industrial and Applied Mathematics, Information Processing Society of Japan |
|
Interests: |
Mixed-Precision Computing, Numerical Linear Algebra, High Performance Computing, Self-Validating Methods |
Curriculum vitae
- 1997
-
1997/10/02
- 生まれました
- 2016
-
2016/04
- 芝浦工業大学 システム理工学部 数理科学科 入学
- 2020
-
2020/03
- 大学卒業 (総代・首席)
- 学士 (数理科学) 取得
2020/04
- 同大学院 理工学研究科 システム理工学専攻 修士課程 入学
- 2022
-
2022/03
- 修士課程 修了 (総代・首席)
- 修士 (システム理工学) 取得
2022/04
- 同大学院 理工学研究科 機能制御システム専攻 博士後期課程 入学
- 日本学術振興会 特別研究員 DC1 採用
- 2024
-
2024/03
- 博士後期課程 修了 (短縮)
- 博士 (工学) 取得
- 日本学術振興会 特別研究員 DC1 中途辞退
2024/04
- 国立研究開発法人理化学研究所 計算科学研究センター 大規模並列数値計算技術研究チーム 特別研究員 採用
- 2025
-
2025/04
- 情報処理学会 論文誌コンピューティングシステム(ACS)編集委員 就任
- 1997
-
October 02, 1997
- Born
- 2016
-
April 2016
- Enrolled in Department of Mathematical Sciences, College of Systems Engineering and Science, Shibaura Institute of Technology
- 2020
-
March 2020
- Received B.S. in Mathematical Sciences
April 2020
- Enrolled in Systems Engineering and Science, Graduate School of Engineering and Science, Shibaura Institute of Technology (Master's Program)
- 2022
-
March 2022
- Received M.S. in Systems Engineering and Science
April 2022
- Enrolled in Functional Control Systems, Graduate School of Engineering and Science, Shibaura Institute of Technology (Doctoral Program)
- Appointed as Research Fellowships for Young Scientists DC1
- 2024
-
March 2024
- Received Ph.D. in Engineering
- Resigned as Research Fellowships for Young Scientists DC1
April 2024
- Appointed as a Postdoctoral Researcher in Large-Scale Parallel Numerical Computing Technology Research Team, RIKEN Center for Computational Science, RIKEN
- 2025
-
April 2025
- Appointed as IPSJ Transactions on Advanced Computing Systems Editorial Committee Member
Recent Works
Apr. 10, 2025
arXiv
This paper addresses emulation algorithms for matrix multiplication. General
Matrix-Matrix
Multiplication (GEMM), a fundamental operation in the Basic Linear Algebra Subprograms
(BLAS),
is typically optimized for specific hardware architectures. The Ozaki scheme is a
well-established GEMM-based emulation method for matrix multiplication, wherein input
matrices
are decomposed into several low-precision components to ensure that the resulting matrix
product
is computed exactly through numerical operations.
This study proposes a novel GEMM-based emulation method for matrix multiplication that
leverages
the Chinese Remainder Theorem.
The proposed method inherits the computational efficiency of highly optimized GEMM
routines
and
further enables control over the number of matrix multiplications, which can enhance
computational accuracy.
We present numerical experiments featuring INT8 Tensor Core operations on GPUs and FP64
arithmetic on CPUs as case studies. The results demonstrate that FP64 emulation using
the
proposed method achieves performance levels of up to 7.4 to 9.8 TFLOPS on the NVIDIA RTX
4090
and 56.6 to 80.2 TFLOPS on the NVIDIA GH200, exceeding the measured performance of
native
FP64
arithmetic. Furthermore, for FP64 computations on CPUs, the proposed method achieved up
to a
2.3x speedup in emulating quadruple-precision arithmetic compared to the conventional
Ozaki
scheme.
Mar. 25, 2025
Journal of Advanced Simulation in Science and Engineering
We propose a method to rapidly generate matrices for real-symmetric eigenproblems. The
proposed
method
produces a reproducible matrix with explicit eigenpairs, where the distribution of the
eigenvalues
can
be controlled by a user. All elements of the generated matrix are rigorous
floating-point
numbers
and
can be represented in simple expressions involving the exact eigenvalues. The exact
eigenpairs
of
the
generated matrix are known in advance; thus, the proposed method contributes to the
validation
of
errors
in approximate eigenpairs. Several constraints on the matrix generated by the proposed
method,
were
produced theoretically.
Jan. 09, 2025
The International Journal of High Performance Computing Applications
This study was aimed at simultaneously achieving sufficient accuracy and high
performance
for
general
matrix multiplications. Recent architectures, such as NVIDIA GPUs, feature
high-performance
units
designed for low-precision matrix multiplications in machine learning models, and
next-generation
architectures are expected to follow the same design principle. The key to achieving
superior
performance is to fully leverage such architectures. The Ozaki scheme, a highly accurate
matrix
multiplication algorithm using error-free transformations, enables higher-precision
matrix
multiplication to be performed through multiple lower-precision matrix multiplications
and
higher-precision matrix additions. Ootomo et al. implemented the Ozaki scheme on
high-performance matrix
multiplication units with the aim of achieving both sufficient accuracy and high
performance.
This paper
proposes alternative approaches to improving performance by reducing the numbers of
lower-precision
matrix multiplications and higher-precision matrix additions. Numerical experiments
demonstrate
the
accuracy of the results and conduct performance benchmarks of the proposed approaches.
These
approaches
are expected to yield more efficient results in next-generation architectures.
Nov. 17-22, 2024
SC24-W
This study proposes a high-performance and reliable eigensolver via mixed-precision
arithmetic
between ordinary and highly-accurate precisions. Eigenvalue decomposition is ubiquitous
in
simulations. Various eigensolvers for computing approximations have been developed thus
far.
If
eigenvalues are narrowly clustered, the computation of eigenvectors may be ill-posed.
Thus,
the
computed eigenpairs may not be sufficiently accurate and lack reliability. In this
study, we
introduce mixed-precision iterative refinement methods to improve the accuracy of
eigenvectors
obtained using numerical methods. This approach contributes to obtaining sufficiently
accurate
results without arbitrary precision eigensolvers. We construct a high-performance and
reliable
eigensolver by combining the iterative refinement methods and EigenExa, a modern
high-performance solver for large-scale and highly parallel computations. Numerical
experiment
results demonstrate the accuracy of the results and performance benchmark of the
proposed
approach.
Public Software
Links